The Easy Guide To Mastering Supply Chain Forecasting
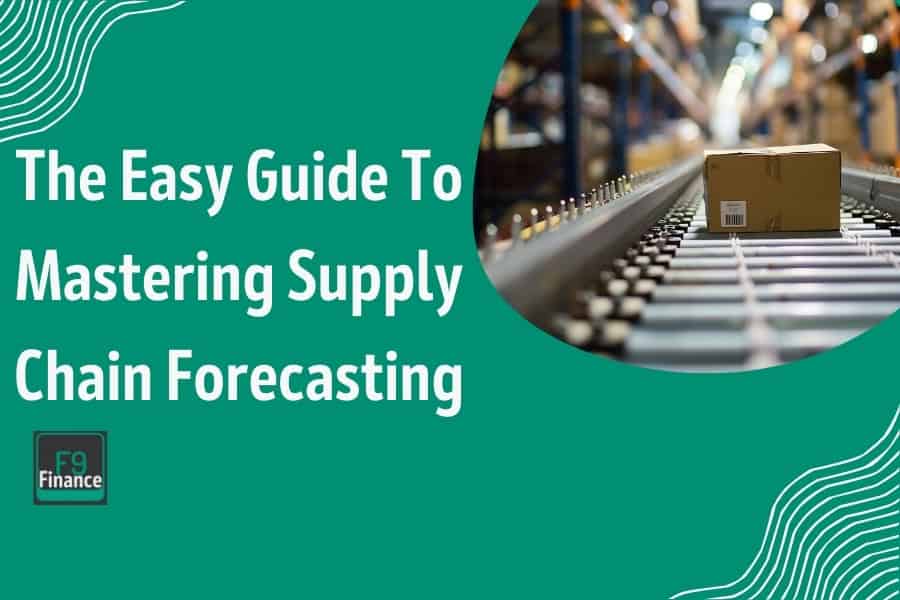
[ad_1]
Accurate supply chain forecasting isn’t just a fancy term to toss around in meetings – it’s the lifeblood of your business. Get it wrong, and you’ll find yourself drowning in overstock or, worse, facing angry customers because you can’t fulfill their orders. But nail it, and it’s like having a GPS that guides you through every twist and turn.
Accurate supply chain forecasting helps you maintain the perfect inventory balance, cuts down waste, optimizes your workforce, and keeps your cash flow steady.
Understanding Supply Chain Forecasting
Alright, let’s break down what supply chain forecasting actually is without making your eyes glaze over. Imagine you’re at a poker table, but instead of cards, you’re dealing with data. Supply chain forecasting is your way of predicting the next move – knowing when to hold ‘em, fold ‘em, and, most importantly, when to stock ‘em.
It’s all about predicting future demand so you can keep your inventory in check, avoid backorders, and make sure you’re not stuck with a warehouse full of stuff no one wants.
Now that we’ve got the basics down, let’s dive into the supply chain forecasting methods behind the madness. Supply chain forecasting can be split into two main camps: qualitative and quantitative methods.
Qualitative Forecasting Methods
- Delphi Method: Picture a group of experts throwing darts at a board – except these darts are their best guesses at future trends. The Delphi Method rounds up opinions from a panel of experts, combines them, and refines them through several rounds until they reach a consensus. Think of it as crowdsourcing for the big leagues.
- Market Research: This one’s pretty straightforward. You hit the streets (or the internet) to gather insights directly from your customers. Surveys, focus groups, interviews – it’s all about getting the lowdown straight from the horse’s mouth.
Quantitative Forecasting Methods
- Time Series Analysis: Fancy name, simple concept. This method looks at historical data to spot patterns and trends over time. It’s like looking in the rearview mirror to predict what’s coming up on the road ahead. If your sales spike every December, time series analysis will help you see it coming.
- Causal Models: These models play detective, looking for cause-and-effect relationships between different factors. For example, if a heatwave always boosts your ice cream sales, a causal model will catch that connection and factor it into your forecast. It’s like having a crystal ball, but one based on hard data.
Why It Matters: The Impact of Supply Chain Management

Alright, here’s the million-dollar question: why should you care about forecasting in supply chain? Let me spell it out for you. When done right, customer demand forecasting can save your bacon in three major ways:
- Inventory Management: No more warehouses crammed with unsellable junk or empty shelves where your hottest items should be. Forecasting helps you strike that perfect balance – keeping enough stock on hand to meet demand without overdoing it.
- Customer Satisfaction: Imagine this – a customer orders something from you, and bam! It arrives on time, exactly as expected. That’s the kind of magic forecasting can work. Happy customers mean repeat business, glowing reviews, and a solid reputation.
- Profitability: This is where it really hits home. Efficient forecasting means you’re not tying up your capital in excess inventory or missing out on sales because you ran out of stock. It’s all about maximizing those profit margins and keeping the cash flowing.
Getting Started with Supply Chain Forecasting
Step 1: Collecting Relevant Data
Alright, let’s kick things off with the backbone of any good forecast – data. Think of data as the fuel that drives your forecasting engine. Without it, you’re just spinning your wheels.
Sources of Data
First up, there’s internal sales data. This is the stuff right under your nose – future sales figures, order histories, and customer preferences. It’s like reading your diary; it tells you exactly what’s been happening in your business.
Next, we have market trends. This is your peek into the crystal ball of industry shifts, consumer behavior, and competitor moves. Ever wonder why everyone started buying fidget spinners out of nowhere? Market trends have the answers.
And then there’s historical data. This is the equivalent of looking at old yearbooks to predict who’s going to be the next prom king or queen. It gives you patterns, seasonality, and those quirky little anomalies that might just repeat themselves.
Importance of Data Accuracy and Completeness
Now, let’s talk accuracy. Imagine you’re baking a cake. If your measurements are off, you’re either getting a pancake or a brick. Same goes for data. Garbage in, garbage out. Inaccurate data will throw your whole forecast off, making you look like a rookie.
Completeness matters too. Picture trying to finish a jigsaw puzzle with half the pieces missing – frustrating and pointless. Make sure you’re covering all bases, gathering data from every nook and cranny of your business. The more complete your data, the clearer your forecast will be.
Step 2: Choosing the Right Forecasting Method
With your data treasure trove ready, it’s time to pick your supply chain forecasting method of choice. Here’s what you need to consider:
Factors to Consider
- Type of Product: Are you dealing with perishable goods or durable items? The nature of your product and the related supply chains affects how you’ll forecast demand.
- Industry Trends: What’s hot and what’s not? Staying tuned into industry buzz can guide you in selecting the method that fits your market dynamics.
- Availability of Data: Got tons of historical data? Great, you might lean towards quantitative methods to predict future sales. Running low on past data? Qualitative might be your friend.
Pros and Cons of Different Methods
Qualitative Methods:
Pros: Great when you don’t have much historical data to work with. Leverages expert opinions and market research.
Cons: Can be subjective and sometimes feel like educated guesswork.
Quantitative Methods:
Pros: Data-driven and often more accurate. Can identify patterns that the human eye might miss.
Cons: Requires substantial historical data. Can be complex and time-consuming to set up.
Implementing Your Forecasting Model
Step-by-Step Walkthrough
Alright, buckle up because we’re about to dive into the nuts and bolts of setting up your forecasting model. This isn’t rocket science, but it’s as close as it gets in the finance world.
- Choosing Your Software: First things first, pick your weapon of choice. Whether it’s Excel, a proprietary software, or some fancy AI-driven tool, make sure you’re comfortable with it. I’ve seen folks spend more time figuring out their software than actually forecasting – don’t be that person.
- Data Collection: You’ve already gathered your data (remember Chapter 2?). Now, it’s time to feed it into your system. Think of it as fueling your car before a road trip – without gas, you’re not going anywhere.
- Configuring Parameters: Set your parameters based on what you want to forecast. Are you looking at monthly sales? Weekly inventory levels? Get specific. This step is like programming your GPS; tell it exactly where you want to go, or you’ll end up lost.
- Running Initial Tests: Before you hit the highway, take a test drive. Run some initial forecasts using historical data to see if your model is spitting out plausible numbers. If it looks like it’s trying to predict the next ice age instead of next month’s sales, you’ve got some tweaking to do.
Inputting Data and Configuring Parameters
Let’s dig deeper into inputting data and configuring those oh-so-important parameters:
- Data Input: Import your historical data into your chosen software. Clean it up – no one likes messy data. Ensure there are no missing values, duplicates, or anomalies unless you’re into surprises.
- Parameter Configuration: Depending on your demand planning method (Time Series, Causal Models, etc.), set your parameters. For Time Series, define your time period (weekly, monthly, yearly). For Causal Models, identify your independent variables that could affect demand (e.g., price changes, marketing campaigns).
How I Implemented a Demand Forecasting Model for a Mid-Sized Retail Business
Let’s get real for a second. Here’s a tale from the trenches of retail finance. I once worked with a mid-sized retail company that was drowning in incorrect forecasts. Their warehouse looked like a scene from “Hoarders” – overstocked in some areas, barren in others.
Step-by-Step Breakdown:
- Data Mining: We started by digging up three years’ worth of sales data. Every transaction, every return, all laid bare.
- Choosing the Tool: We went with a robust forecasting tool that integrated well with their existing ERP system.
- Setting Parameters: We focused on monthly sales forecasts, tailoring parameters around seasonal trends, promotions, and economic factors.
- Initial Test Runs: We ran demand forecasts on past data to check accuracy. There were hiccups – the first runs predicted Halloween costume sales in February. But after tweaking, we got it right. We also validated with the sales team to make sure it made walking around sense.
- Implementation: Once confident, we rolled out the model. Within six months, stockouts were down by 30%, and profitability was up by 15%. The warehouse was finally back to looking like a place of business, not a post-apocalyptic storage unit.
Overcoming Common Challenges
Implementing a forecasting model isn’t all rainbows and unicorns. Here are some common pitfalls and how to dodge them:
Dealing with Data Discrepancies
- Identify the Issue: Discrepancies often come from inconsistent data entry. Run validation checks regularly.
- Cleaning Up: Use data-cleaning tools to hunt down and fix outliers or errors. Treat this data like you would raw chicken – clean it thoroughly before use.
Adjusting for Seasonality and Market Fluctuations
- Seasonality: Incorporate seasonality indices into your model. If your sales spike every December, your model should know that supply chains need to adjust in November.
- Market Fluctuations: Keep an eye on market trends and adjust your model parameters accordingly. Flexibility is key – rigid models break easily under pressure.
Analyzing and Interpreting Forecasts
So, you’ve put in the legwork, gathered your data, and set up your forecasting model. Now comes the part where we turn those numbers into something you can actually use. Buckle up, because we’re diving into the world of analyzing and interpreting forecasts. This is where the magic happens – or where it all falls apart if you’re not careful.
Making Sense of the Results
Alright, let’s make sense of this chaos. You’ve got a bunch of forecasts staring back at you – now what? Here’s how to cut through the noise and find the gold.
Key Metrics to Track
- Demand Forecasting Accuracy: This is your bread and butter. How close is your supply chain forecast to reality? You’ll measure this by comparing your forecasted values against actual outcomes. The closer, the better.
- Error Rates: No one’s perfect, and neither is your forecast. Track metrics like Mean Absolute Error (MAE) or Mean Squared Error (MSE) to see where things went off the rails. Lower error rates mean you’re on the right track.
- Bias: Is your forecast consistently over or under-predicting? That’s bias, my friend. If you’re always overshooting, it’s time to recalibrate.
- Tracking Signal: This nifty metric tells you if your forecast is systematically off in one direction. It’s like having a compass that points out when you’re veering off course.
Supply Chain Forecasting Important Tools and Software for Analysis
Let’s talk gadgets and gizmos. You don’t have to be a tech whiz to get this right – just know which tools to wield.
- Excel: Old faithful. With its built-in functions and add-ons like Solver, Excel is your go-to for quick and dirty analyses.
- R or Python: If you’re ready to get your hands dirty with some code, these programming languages offer powerful libraries for advanced forecasting and analysis. Think of them as the Swiss Army knives of data science.
- Specialized Software: Tools like SAP IBP, Oracle Demantra, or even more niche solutions like JDA offer robust platforms specifically designed for supply chain forecasting and predicting demand. These are your high-end sports cars – great if you know how to drive them.
Adjusting Strategies Based on Forecasts
You’ve crunched the numbers, now it’s time to tweak your game plan. Here’s how you turn those insights into action.
How to Tweak Your Supply Chain Operations Based on Forecast Data
- Inventory Adjustments: If the forecast shows a spike in demand next month, ramp up production or stock up on raw materials. If it predicts a lull, scale back to avoid overstock.
- Workforce Planning: Use your forecasts to plan staffing needs. Busy season ahead? Bring in extra hands. Slow period? Maybe it’s time to schedule that training everyone’s been putting off.
- Supplier Coordination: Keep your suppliers in the loop. Share your supply chain forecasts with them to ensure they’re prepared to meet your demands without delays.
- Marketing and Sales Alignment: Align your marketing campaigns with your supply forecasts. If you know demand will peak, push those ads hard. Expecting a drop? Focus on retention strategies to keep your current customers engaged.
Case Study: A Success Story
Let’s dive into a real-life example to see how this plays out.
Imagine a mid-sized electronics retailer we’ll call “ZapTech.” ZapTech was notorious for stockouts during major product launches – think new smartphone releases. Customers were frustrated, and sales were lost. Enter robust forecasting.
ZapTech implemented demand forecasting techniques including a time series forecasting model using three years of historical sales data. They incorporated external factors like tech trends and competitor launches. With these insights, they adjusted their supply chains and inventory levels well before the next big launch.
The results? Stockouts dropped by 40%, customer satisfaction soared, and sales during launch periods increased by 25%. By leveraging accurate forecasts, ZapTech turned chaos into a symphony of efficiency.
Continuous Improvement and Adaptation
Here’s the deal: your supply chain forecasting is only as good as its last update. Regular check-ups are a must if you want to stay ahead of the curve.
Regularly Reviewing and Updating Forecasts
Think of your forecasts like your car – they need regular maintenance. Set up a schedule to review and update your forecasts. Monthly, quarterly, heck, even weekly if you’re in a volatile market. The point is, stale data equals bad decisions.
Using Feedback Loops to Improve Accuracy
Feedback loops are your new best friend. After each period, compare your forecast against actual outcomes. Where did you nail it? Where did you miss the mark? Use this intel to fine-tune your model. It’s like learning from your mistakes, but with numbers instead of life regrets.
Incorporating New Data and Market Conditions
New data is like fresh intel on your competition – invaluable. Incorporate the latest sales figures, market trends, and any other relevant info into your model. Don’t let your forecast become yesterday’s news. Adapt your parameters and input fresh data regularly to keep your predictions sharp.
Being Agile in the Face of Unforeseen Disruptions
Let’s face it, sometimes the universe throws a curveball – economic downturns, supply chain hiccups, global pandemics (yep, we’ve been there). Your job? Stay agile. When chaos hits, quickly reassess your forecasts and adjust. Pivot faster than a startup in Silicon Valley if you need to.
Have any questions? Are there other topics you would like us to cover? Leave a comment below and let us know! Also, remember to subscribe to our Newsletter to receive exclusive financial news in your inbox. Thanks for reading, and happy learning!
[ad_2]