Start a New Year of Learning on the Right Foot | by TDS Editors | Jan, 2025
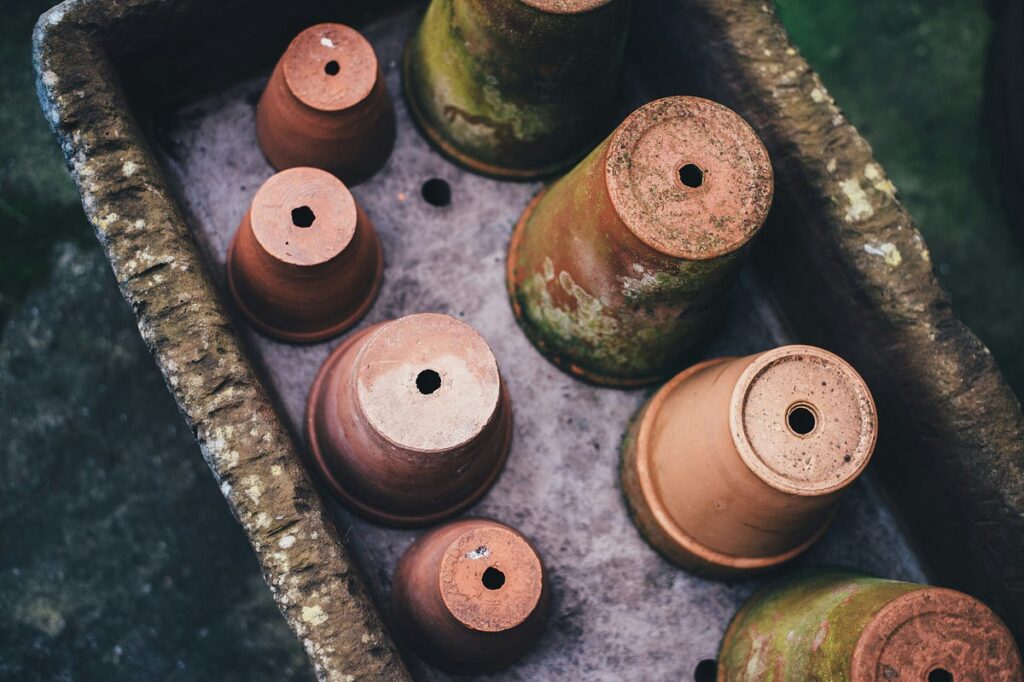
[ad_1]
Feeling inspired to write your first TDS post? We’re always open to contributions from new authors.
Happy new year! Welcome back to the Variable!
The ink has barely dried on our 2024 highlights roundup (it’s never too late to browse it, of course), and here we are, ready to dive headfirst into a fresh year of learning, growth, and exploration.
We have a cherished tradition of devoting the first edition of the year to our most inspiring—and accessible—resources for early-stage data science and machine learning professionals (we really do!). We continue it this year with a selection of top-notch recent articles geared at beginner-level learners and job seekers. For the rest of our readers, we’re thrilled to kick things off with a trio of excellent posts from industry veterans who reflect on the current state of data science and AI, and share their opinionated, bold predictions for what the year ahead might look like. Let’s get started!
2025: Ready, Set, Go!
Data science and machine learning, step by step by step
- The Essential Guide to R and Python Libraries for Data Visualization
With or without AI, charts and plots aren’t going anywhere anytime soon. Sarah Lea maps out the key libraries in which current and aspiring data scientists should gain fluency. - Roadmap to Becoming a Data Scientist, Part 2: Software Engineering
Programming isn’t going anywhere in 2025, either. Vyacheslav Efimov’s guide outlines the coding essentials that will lead you to data science success. - Missing Data in Time-Series: Machine Learning Techniques
One constant trait of real-world data: it’s messy! Learn how to navigate the chaos by following along Sara Nóbrega’s primer on handling missing data. - Causality — Mental Hygiene for Data Science
Taking a few steps back from the more nitty-gritty aspects of data science work, Eyal Kazin’s recent deep dive constitutes a “gentle intro” to the intricate art of detecting, interpreting, and applying causality. - Master Machine Learning: 4 Classification Models Made Simple
For anyone who enjoys structure and clarity above all else, Leo Anello’s (extremely) thorough, 15-step tutorial on classification models would be a perfect starting point from which to expand your ML know-how. - 2024 Survival Guide for Machine Learning Engineer Interviews
Whether you’re already applying for your first MLE job or contemplating it as one of your goals for the year, don’t miss Mengliu Zhao’s “survival guide,” aimed specifically at junior-level practitioners. - Machine Learning Basics I Look for in Data Scientist Interviews
Tackling the occasionally opaque hiring process from the other end of the table, Farzad Nobar created a helpful resource to help job applicants zoom in on the topics that really matter to employers. - 100 Years of (eXplainable) AI
Beyond the whats and hows of day-to-day work, there are also the whys: why did this model produce these outputs? Sofya Lipnitskaya’s explainer unpacks the history of AI explainability in the context of the recent rise of LLMs. - How to Build a General-Purpose LLM Agent
To end on a more hands-on note—and to satisfy the curiosity of all of you who’ve heard the buzz around AI agents—we highly recommend Maya Murad’s step-by-step guide, which can form “the groundwork for designing your own custom agentic architecture” down the line.
[ad_2]