Demonstrating Prioritization Effectiveness in Sales | by Gonzalo Espinosa Duelo | Jun, 2024
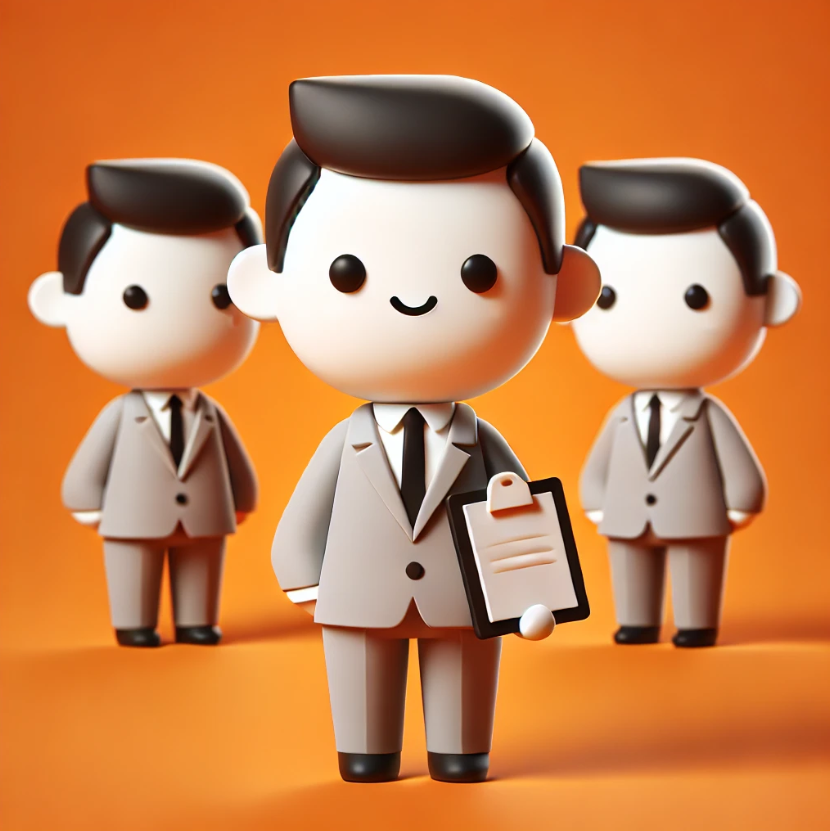
[ad_1]
Let’s imagine two companies in the B2B/B2C context, who are direct competitors and of the same size. Both companies have their own sales team repeating daily a sales process for inbound leads, but they use a radically different sales strategy.
Their processes are the following:
- At Company A, the sales team starts their morning by calling the most recent leads, convinced that these fresh contacts are their best bet.
- At Company B, the scene is quite different. This team begins their day armed with data-driven insights. They’ve invested in a Predictive Lead Scoring prioritization system that analyzes a variety of factors — from user profile to engagement history.
What are your thoughts? Which approach do you think will be more effective in prioritizing leads?
After working for several years on the implementation of prioritization algorithms, I’ve compared dozens of different systems across various sectors.
In today’s sales context, companies spend a lot of resources on SDRs or sales agents for initial outreach and lead qualification. They often lack precise methodologies to identify the most promising leads and simply work all of them without any prioritization.
Most agents prioritize leads based on their own human criteria, which is often biased by personal and non-validated perspectives. Conversely, among the few that implement prioritization methods, the predominant strategy is based on ‘fresh-contact’ criteria, which is still very rudimentary.
This fact blows my mind in the middle of the era of AI, but sadly, it is still happening.
Drawing from practical insights as a Lead Data Scientist in developing Predictive Lead Scoring systems across different sectors, I can state that companies that adopt these technologies reduce operational costs by minimizing work on poorly qualified leads, thereby improving their ROI significantly.
Moreover, by improving efficiency and effectiveness in lead management, they become more precise about determining the prospect’s timeframe for making a decision and drive higher revenue growth.
I’ve observed that companies adopting correctly Predictive Lead Scoring have seen conversion increases of more than 12%, reaching over 300% in some cases.
Addressing this critical need, this article discusses the benefits of taking advantage of a Predictive Lead Scoring model as a prioritization system compared to traditional strategies, as well as the most effective actions to maximize conversion using these methods.
As always, I will support my statement with real data.
The following plot shows a comparison of the conversion gain in a company using only the “Most-Fresh” strategy against a “Predictive Lead Scoring” prioritization.
The analysis was conducted with a real business case, involving 67k contacts (in which 1500 converted to customers) from a B2C company.
The gain is represented by exploring the reached conversion for a particular percentage of leads worked, sorted by the prioritization criteria.
For the methodologies exposed above, their performance are as follows:
The black line represents the random prioritization, providing 50% conversion for 50% of leads worked.
The “most-fresh” strategy offered a slightly better performance than do it randomly, presenting 58% of conversion for 50% of leads processed.
In contrast, the Machine Learning approach achieved an impressive 92% of conversions with just 50% of leads processed.
While the “most-fresh” method offered a similar random performance, the Predictive Lead Scoring showcased much better prioritization.
Notice that Predictive Lead Scoring achieved an impressive Pareto effect by reaching 81% of conversions with only 30% of leads processed.
Arriving at this point, it has been demonstrated that Company B will provide better results than Company A.
Company A assumed that their leads with recent interest, were the best performing leads. They believed that recent interest suggested they were currently considering a purchase. However, this may not be the case.
A recent lead might be curious, but not necessarily ready to make a purchase.
Some leads might fill out a form or sign up out of casual interest, without any real intention to buy. Conversely, others who may not have contacted recently could have a stronger ongoing need for the product or service.
Company B considered additional relevant factors, like user profile, past engagement, buying signals and behavioral indicators, all integrated in one tool.
Their Predictive Lead Scoring also examined lead recency, but instead of relying solely on this element, it was viewed as an extra signal that may be considerably or more relevant depending on the lead profile.
This data-driven approach allowed them to prioritize leads with the highest potential for conversion, rather than just the most recent ones.
By leveraging Predictive Lead Scoring, they are able to effectively identify and focus on leads that are more likely to convert, thereby maximizing their sales efficiency and overall conversion rates.
In summary, whereas Company A assumed that recency is the unique characteristic that equates to interest, Company B’s data-driven approach gave a more refined and effective strategy for lead prioritizing and conversion.
[ad_2]