The Easy Way To Start Using Statistical Forecasting
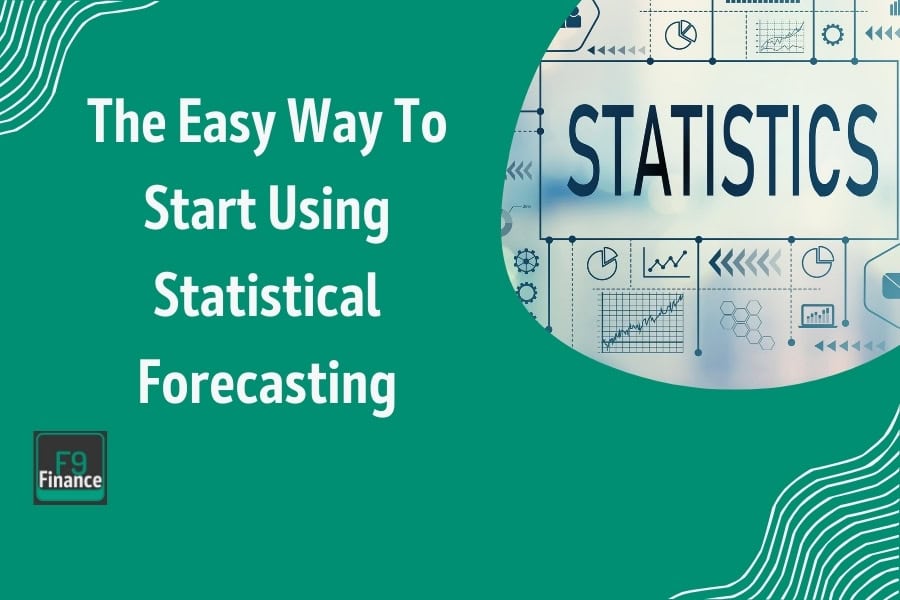
[ad_1]
Statistical forecasting is all about using historical data to predict future outcomes.
Think of it as the financial version of a crystal ball, minus the mystical mumbo jumbo. At its core, it involves analyzing past trends and patterns to make educated guesses about what lies ahead. This isn’t a new concept; it’s been around for quite some time, evolving alongside the financial industry.
Back in the day, you could find early forms of forecasting in simple trend analysis and seasonal adjustments, but today, with tech advancements, it’s become an essential tool—almost like a financial GPS guiding strategic decisions.
So, why should we care about statistical forecasting?
With markets fluctuating faster than my coffee consumption on a Monday morning, having a reliable forecasting method is like having an ace up your sleeve. It’s not just about predicting the next big market shift; it’s about making informed decisions based on data rather than gut feelings.
Whether it’s planning budgets, managing risks, or guiding investment strategies, statistical forecasting empowers us to cut through the noise and make sense of the chaos. By integrating forecasting into our decision-making process, we’re not just reacting to changes but proactively shaping our financial future by accurately predicting future demand. That’s why it’s a game-changer in the world of finance.
How Statistical Forecasting Is Used In Finance
Let’s dive into why statistical forecasting is the unsung hero in finance, making life a tad easier for those of us navigating the turbulent financial seas.
Demand history plays a crucial role in financial forecasting, as it provides the historical data patterns necessary for time series methods to project future values and reduce uncertainty.
Strategic Financial Planning
You know, when it comes to budgeting and plotting out financial strategies, forecasting is like having a map in a treasure hunt. It helps us anticipate revenues and expenses, ensuring that our financial plans aren’t just optimistic fantasies but grounded in reality.
Understanding the causal factors, such as prices, promotions, and economic indicators, can enhance financial planning by revealing the relationships between these variables and our financial outcomes.
With precise forecasts, I can allocate resources more effectively, tweak budgets with a degree of accuracy that would make a Swiss watch jealous, and ultimately, ensure the financial health of the organization. It’s all about being prepared for what’s around the corner, rather than being caught off-guard.
Risk Management Using Historical Data
This is where forecasting truly shines as a preventative measure. By using statistical methods to analyze past data and current trends, we can pinpoint potential risks before they become full-blown problems.
It’s like having a weather app that warns us about impending storms, allowing us to batten down the hatches in advance. This proactive approach helps in mitigating financial losses and safeguarding assets. Forecasting gives us the foresight to make adjustments, reallocate funds, or even rethink strategies to cushion the blow of potential financial downturns.
Investment Analysis
In the world of investing, where fortunes are made and lost faster than you can say “diversification,” forecasting offers a semblance of predictability.
By leveraging statistical forecasts, I can make informed investment decisions, spotting trends that could indicate lucrative opportunities or red flags that scream “proceed with caution.” It’s not foolproof, but it certainly beats playing a game of financial Russian roulette. Forecasts help refine investment strategies, ensuring that decisions are backed by data rather than just gut instincts or wishful thinking.
Key Concepts For Statistical Forecasting

Diving into the world of statistical forecasting can sometimes feel like you’ve stumbled into a mix of alphabet soup and math club jargon. Statistical techniques play a crucial role in forecasting by leveraging historical data to predict future behaviors, ensuring accuracy across different time periods and spatial resolutions. But fear not—we’re here to decode the lingo and make it all make sense.
Understanding the Jargon
Imagine you’re at a dinner party where everyone’s throwing around terms like “time series” and “regression,” and you’re nodding along while thinking, “What the heck are they talking about?” Well, a time series is simply a series of data points listed or graphed in time order. It’s like keeping track of your coffee consumption throughout the week to spot trends.
Regression, on the other hand, is a bit like connecting the dots on a scatter plot to see if there’s a relationship between two variables—say, how much coffee you drink and your productivity levels. These are just two of the many terms you’ll encounter, but understanding them is key to navigating the forecasting landscape.
Statistical Forecasting Methods: Linear Regression

Some popular statistical forecasting techniques include moving averages and exponential smoothing.
Moving averages are like a rolling average that helps smooth out fluctuations and highlight trends over time—imagine it as averaging your coffee intake over a month for a clearer picture.
Exponential smoothing gives more weight to recent observations, making it particularly useful when you expect recent trends to continue. It’s like paying more attention to this week’s caffeine highs and lows to predict tomorrow’s buzz.
Building a Forecasting Model
Creating a forecasting model might sound like a daunting task, but breaking it down into manageable steps can make it much more approachable. Here’s how you can build your own forecasting model:
- Data Collection: Start by gathering all relevant historical data. This is your foundation, so the more accurate and comprehensive your data, the better.
- Data Analysis: Dive into the data to identify patterns, trends, and correlations. This step is like detective work, uncovering the hidden stories within your data.
- Model Selection: Choose a statistical model that fits your data and the problem you’re trying to solve. Whether it’s a simple moving average or a complex ARIMA model, the right choice is crucial.
- Model Estimation: Use your historical data to estimate the parameters of your chosen model. This step fine-tunes your model to reflect the nuances of your data.
- Model Validation: Test your model to ensure it performs well. Techniques like cross-validation can help you compare predicted outcomes with actual results.
- Model Deployment: Finally, put your model to work in a real-world setting. Use it to generate forecasts and inform your strategic decisions.
By following these steps, you can transform raw data into powerful forecasts that guide your decision-making.
Step-by-Step Guide to Implementing Statistical Forecasting
Embarking on the journey of statistical forecasting can be daunting, but fear not! I’m here to guide you through it step by step, turning what might seem like a math maze into a manageable path.
Step 1: Preliminary Steps
Before diving into the numbers, it’s essential to set the stage.
First, identify your goals. What are you hoping to achieve with your forecasting? Whether it’s improving budget accuracy or predicting sales trends, having clear objectives will guide your process. Next, gather your data. Think of this as collecting all the puzzle pieces; the quality of your data will directly impact your forecasts. Ensure that your data is relevant, comprehensive, and as up-to-date as possible.
Step 2: Choosing the Right Method
With your goals and data in hand, it’s time to choose the right forecasting method. This decision depends on factors such as the nature of your data, the time horizon of your forecast, and the complexity you’re willing to handle.
For example, if you’re dealing with a lot of historical data and seeking a longer-term forecast, quantitative methods like time series analysis might be your go-to. Conversely, if you’re working with limited data, a qualitative approach could be more suitable.
Step 3: Data Analysis and Model Development
Now, let’s roll up our sleeves and get into data analysis and model development. Start by cleaning your data—remove outliers, fill in missing values, and ensure consistency. This step is crucial, as clean data is the foundation of reliable forecasts.
Once your data is prepped, begin your analysis. Develop your model by identifying patterns, trends, and relationships within the data. This is where the magic happens—turning raw data into insightful forecasts.
Step 4: Testing and Validation
With your model built, it’s time to put it to the test. Testing and validation ensure your forecasts are accurate and reliable. Use a portion of your data to validate your model, comparing predicted outcomes with actual results. If the model doesn’t perform well, tweak and refine it. This step is all about iterating to get the most dependable results.
Step 5: Implementation and Monitoring
Finally, implementation and monitoring bring your forecasts to life. Apply your forecasts in real-world scenarios, such as informing strategic decisions or adjusting operations. But don’t stop there—monitor their performance continuously. Track how well your forecasts align with real outcomes and be prepared to adjust your model as needed. This dynamic approach helps maintain accuracy and relevance over time.
Case Studies
Let’s dive into some real-life examples of statistical forecasting in action. These case studies will show how forecasting isn’t just a theoretical exercise but a practical tool that can transform business operations.
Case Study: Forecasting in a Retail Company Using Exponential Smoothing

Picture this: a bustling retail company aiming to optimize its inventory management to reduce costs and enhance customer satisfaction. The goal was clear—cut down on excess stock while ensuring popular items never hit the dreaded “out of stock” status. Here’s how they did it:
- Data Collection: The company started by gathering historical sales data, seasonal trends, and customer demand patterns. This wealth of information set the foundation for their forecasting model.
- Method Selection: After reviewing their data, they decided on a time series analysis, as it offered the best fit for analyzing sales trends over time.
- Model Development: They developed a model that accounted for seasonality and promotional events, which are big drivers in retail. This nuanced approach allowed them to forecast sales with impressive accuracy.
- Testing and Validation: The model was tested using past data, refining it until the forecasts closely matched actual sales figures.
- Implementation: With a reliable model in place, the retail company adjusted its inventory management strategy. They started ordering stock based on their forecasts, resulting in fewer overstocks and stockouts.
Results: The outcome was a significant reduction in holding costs and improved customer satisfaction, as products were available when and where customers wanted them. This successful application of statistical forecasting highlighted how data-driven insights could lead to more efficient operations.
Case Study: Financial Services Firm
Now, let’s shift gears to a financial services firm looking to enhance its risk management strategy through forecasting. This firm wanted to anticipate market shifts and adjust its portfolio accordingly.
- Data Gathering: The firm collected extensive market data, including interest rates, stock prices, and economic indicators.
- Choosing the Technique: They opted for regression analysis to understand how different market variables could influence future trends.
- Model Crafting: The team built a model that captured the relationships between these variables, allowing them to predict potential market movements.
- Validation Process: The model was rigorously tested, comparing forecasts with historical market changes to ensure reliability.
- Strategy Execution: Armed with their forecasts, the firm adjusted its investment strategies, reallocating assets to mitigate potential risks and seize upcoming opportunities.
Lessons Learned: The forecasting model proved invaluable in identifying potential downturns and optimizing investment decisions. However, the firm learned that while forecasts provided a strategic edge, they needed to be part of a broader, flexible strategy that could adapt to unforeseen market events.
Overcoming Common Challenges
When it comes to statistical forecasting, challenges are part of the journey. But with the right strategies, they can be transformed into opportunities for improvement. Here’s how we tackle the most common hurdles in the world of forecasting.
Dealing with Data Limitations
Data limitations are like trying to complete a puzzle with missing pieces. It’s frustrating but manageable with the right approach. Start by identifying the gaps in your data and determine their impact on your forecasting model.
One effective strategy is to use data interpolation techniques to estimate missing values, or leverage external data sources to fill in the blanks. Additionally, focus on data quality over quantity; sometimes, it’s better to work with a smaller, cleaner dataset than a large, messy one. Regular audits and data cleaning can also go a long way in maintaining the integrity of your data.
Handling Uncertainty and Volatility
In the finance world, uncertainty and volatility are like the uninvited guests who crash your party—unpredictable and often unwelcome. To improve forecast accuracy amidst such conditions, consider adopting a range of forecasting models.
This approach, known as ensemble forecasting, combines different models to provide a more robust prediction. Additionally, scenario analysis can help by providing a framework to evaluate various possible future states and their impacts. Always be prepared to adapt your forecasts as new data becomes available; flexibility is key in navigating uncertainty.
Overcoming Implementation Barriers
Integrating forecasting into existing financial systems can feel like trying to fit a square peg into a round hole. The trick is to focus on alignment and gradual integration. Start by ensuring that the forecasting tools and techniques you choose are compatible with your current systems. Involve IT and finance teams early in the process to address technical and operational challenges.
Training is crucial—equip your team with the skills needed to effectively use forecasting tools and interpret results. Finally, foster a culture that values data-driven decision-making, ensuring that forecasting becomes a core component of your strategic processes.
[ad_2]