How To Solve Common Issues With Financial Data Quality
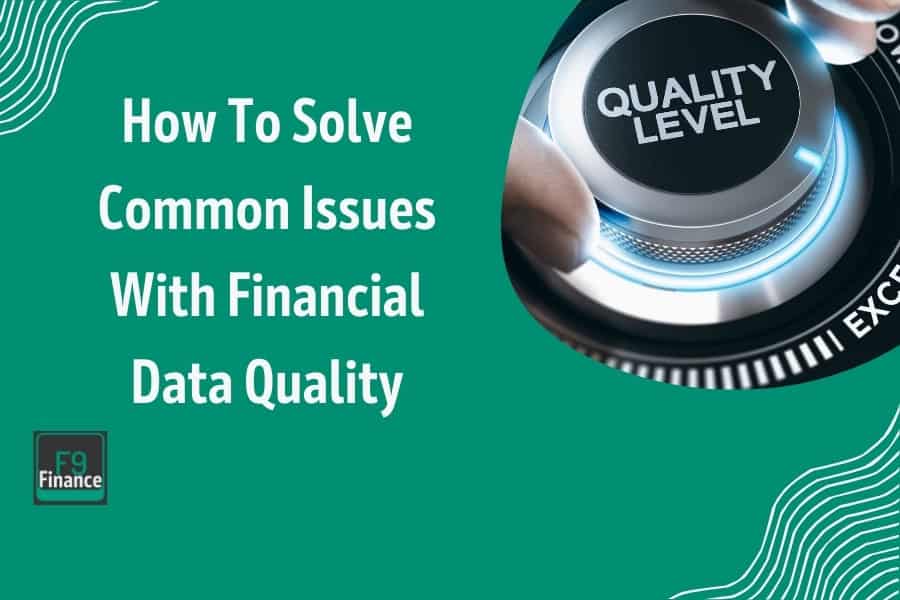
[ad_1]
Having spent years in the trenches of financial analysis, I can tell you firsthand that data quality is the unsung hero of good decision-making. It’s the difference between a forecast that hits the mark and one that misses by miles due to data quality problems.
I remember a project a few years back where we were knee-deep in quarterly projections. Everything was lined up for a stellar presentation to the board, but a last-minute review exposed a glaring error: duplicate entries had inflated our revenue figures.
Panic ensued, presentations were rewritten, and there were a few too many caffeine-fueled nights. That near-disaster taught me a valuable lesson: the devil is indeed in the details, and poor data quality can throw a wrench in the best-laid plans.
The Stakes: What’s Really at Risk
Now, let’s talk about the stakes. Poor data quality isn’t just a matter of embarrassment or a few extra late nights. It has real consequences. Imagine making investment decisions based on faulty data—it’s like building a house on quicksand. The financial repercussions can be enormous, from missed opportunities and wasted resources to regulatory penalties and damaged reputations.
When data quality slips, the ripple effect can be felt throughout the organization, affecting everything from compliance to customer trust. Data consumers rely on high-quality data for analysis and decision-making, making it crucial to ensure data quality and accessibility. Simply put, maintaining high data quality is not just a best practice; it’s a necessity for safeguarding the financial health and integrity of any operation. So, as we dive deeper into the nitty-gritty of financial data quality, keep in mind that this isn’t just about spreadsheets and figures—it’s about creating a solid foundation for informed, strategic decision-making.
Understanding Financial Data Quality

Defining Data Quality in Finance
In finance, data quality hinges on four key attributes: accuracy, completeness, consistency, and timeliness.
Accuracy ensures that the numbers reflect reality—a critical factor when you’re making high-stakes financial decisions. Completeness means no missing pieces; every data point should be accounted for to provide a full picture. Consistency involves uniformity across data sets so that reports and analyses align without contradiction. Lastly, timeliness ensures that data is up-to-date and relevant, allowing for decisions based on the latest available information. Monitoring these attributes through data quality metrics is essential for identifying trends and areas needing improvement.
I remember one instance where data discrepancies nearly led to a costly error. We were preparing a financial report for a major client, and everything seemed perfect—until we noticed that some figures were misaligned due to inconsistent data entries from different sources. It was a glaring discrepancy that, if left unchecked, could have led to incorrect reporting and a potential breach of trust with the client. This incident was a wake-up call about the importance of vigilance in maintaining data quality.
Why It’s Complicated: The Layers of Data Quality
Managing data quality isn’t just about ticking boxes on a checklist. It’s a multi-layered process that involves navigating complex systems and aligning various data sources. One of the main challenges is the sheer volume of data that organizations deal with today. With information pouring in from multiple channels, ensuring accuracy and consistency becomes a daunting task.
Moreover, financial data isn’t static—it evolves, which means continuous monitoring and updates are crucial. Another complexity is the integration of new technologies and data systems, which can sometimes lead to compatibility issues or data silos, where information is trapped in one part of the organization and inaccessible to others who need it.
These layers of complexity require a robust framework for managing data quality—a task easier said than done. Data quality tools are essential instruments that ensure data accuracy, consistency, and reliability, while also helping organizations comply with standards and regulations. It involves not just implementing the right tools, but also fostering a culture that prioritizes data integrity. It’s a challenging but essential journey, ensuring that financial operations remain reliable and decisions are made on a solid foundation.
Common Issues in Financial Data Quality
Incomplete or Missing Data: The Silent Saboteur
In the whirlwind of financial reporting, incomplete or missing data is like a silent saboteur lurking in the shadows. It often creeps in due to human error, system glitches, or fragmented data collection processes. When pieces of data are missing, it’s like trying to complete a jigsaw puzzle with half the pieces—you’re left with gaps that can distort the big picture.
The implications are serious. Incomplete data can lead to flawed financial reports, skewing results and potentially leading to misguided strategic decisions. Imagine evaluating a potential investment with only half the relevant data—you might end up making a decision based on skewed insights, which could be costly. This issue underscores the importance of thorough data validation and checks to ensure that every data point is accounted for.
Inaccurate Data: When Numbers Just Don’t Add Up
Inaccuracy in financial data is a deal-breaker. These inaccuracies often stem from manual data entries, outdated systems, or lack of standardized data protocols, which undermines data consistency. It’s like playing a game of telephone—by the time the message reaches the end, it’s completely different from the original.
When numbers don’t add up, it can lead to incorrect budgeting, forecasting errors, and ultimately, financial losses. For instance, if revenue figures are inflated due to inaccuracies, a company might overspend based on projected profits that don’t actually exist. Precision is paramount in finance, and ensuring data accuracy is a non-negotiable standard.
Data Duplication: Seeing Double
Duplicate data entries are like seeing double—it can make you question your sanity and the validity of your reports. These duplicates often arise from multiple entries in different systems or lack of integrated data management protocols. Maintaining accurate and comprehensive customer data is crucial for ensuring data quality and integration across various applications.
The presence of duplicate data can significantly skew analyses, leading to erroneous conclusions. For example, if a client’s purchase is counted twice, it artificially inflates sales figures, misleading stakeholders about the company’s actual performance. This issue highlights the necessity for a centralized data system that ensures each entry is unique and unrepeatable.
Inconsistent Data: When Information Doesn’t Match Up
Inconsistencies in data are akin to reading conflicting stories in different newspapers about the same event—confusing and unreliable. This issue often occurs when data is pulled from various sources or platforms that don’t communicate effectively.
For instance, if sales data from a retail store doesn’t match the figures in the accounting system, it raises red flags and could indicate deeper systemic issues. Inconsistent data can impact decision-making, leading to mistrust in data-driven strategies. Ensuring consistency requires robust data integration practices and regular cross-platform audits to ensure all systems are aligned and speaking the same language.
Real-Life Examples of Data Quality Issues
Case Study 1: The Cost of Inaccurate Forecasting
Picture this: a bustling tech company on the brink of launching its latest product, with excitement running high across the boardroom. Forecasts painted a rosy picture, predicting a surge in demand and revenue. But beneath the surface, the data driving these forecasts was flawed—riddled with inaccuracies from inconsistent data sources and outdated figures.
As the product hit the market, it became painfully clear that the predictions were way off. Sales fell short, inventory piled up, and what was supposed to be a triumph turned into a financial quagmire. The root cause? Inaccurate data had led to overoptimistic forecasts, resulting in overproduction and significant financial losses. This case underscores the critical importance of data accuracy in forecasting—where even a small discrepancy can spiral into major fiscal repercussions.
Case Study 2: The Impact of Incomplete Data on Compliance
Let’s shift gears to a financial institution grappling with compliance regulations. In the fast-paced world of finance, regulatory compliance is non-negotiable, with strict requirements for data reporting. However, this institution faced a significant challenge: missing data points within their compliance reports due to fragmented data collection processes.
The oversight didn’t go unnoticed for long. Regulatory bodies slapped the institution with hefty penalties, citing incomplete data submissions. The financial hit was compounded by the damage to the institution’s reputation in the market. This scenario highlights the peril of incomplete data—where missing pieces can lead to compliance breaches and costly penalties. It serves as a stark reminder that data completeness isn’t just a procedural nicety; it’s a critical component of maintaining regulatory compliance and safeguarding an organization’s standing in the industry.
Step-by-Step Solutions to Improve Data Quality
Step 1: Conducting a Data Quality Assessment
Before diving into solutions, it’s crucial to know where the problems lie. Conducting a thorough data quality assessment is like getting a full diagnostic on your car before a road trip.
Start by identifying key data sets and scrutinizing them for errors—look for common culprits like inaccuracies, incompleteness, and inconsistencies. Prioritize these issues based on their impact on your financial operations and decision-making processes. This assessment is the foundation for any remediation efforts, helping you focus on the most critical areas that need immediate attention.
Step 2: Establishing Data Governance
Once the problem areas are clear, it’s time to establish a robust data governance framework. Think of this as setting the rules of the road for your data highway. Begin by defining roles and responsibilities—who owns the data, who can access it, and who is responsible for maintaining its quality.
Develop policies and standards that ensure data is collected, stored, and utilized consistently across the organization. This framework acts as a guardian, maintaining the integrity and reliability of your data over time.
Step 3: Implementing Data Cleaning Processes
With governance in place, roll up your sleeves and start cleaning!
Data cleaning involves scrubbing your datasets to remove errors and standardize information. Use techniques like deduplication to eliminate double entries and validation checks to ensure data accuracy. Standardization processes help align data formats, making it easier to merge information from different sources. These cleaning processes are essential for transforming raw, messy data into a polished, reliable asset for your organization.
Step 4: Continuous Monitoring and Improvement
Data quality isn’t a one-and-done task; it requires ongoing vigilance. Set up systems for continuous monitoring to catch and address issues as they arise. Implement automated tools that can alert you to anomalies and discrepancies in real-time. Regular audits and reviews can help ensure that your data governance policies are being followed and that your data remains accurate, complete, and consistent. This commitment to continuous improvement ensures that your data quality keeps pace with the evolving needs of your business.
Tools and Technologies for Data Quality Management
Overview of Popular Tools
Navigating the sea of data quality management can feel overwhelming, but luckily, there are some trusty tools that can steer you in the right direction. Let’s dive into a few of the leading solutions designed to keep your data ship shape:
- Talend Data Quality: Talend is like your Swiss army knife for data quality management. It offers a comprehensive suite of functionalities, from profiling and cleansing to monitoring and enrichment, making it a top pick for businesses looking to streamline their data processes.
- Informatica Data Quality: Renowned for its robust data profiling and cleansing capabilities, Informatica ensures your data remains accurate and consistent. It’s particularly praised for its integration features, which allow seamless data management across platforms.
- IBM InfoSphere QualityStage: If you’re dealing with large volumes of data, IBM’s tool is designed to handle the heavy lifting. It excels in data standardization and matching, helping you maintain a clean and reliable database.
- SAP Data Services: Offering strong data integration and cleansing features, SAP Data Services is a favorite among enterprises aiming for high-quality, actionable insights. Its ability to handle complex data transformations makes it a solid choice for businesses of all sizes.
These tools are your allies in the quest for pristine data, each bringing its unique strengths to the table.
How Technology Can Help: Data Quality in the Age of AI
Now, let’s talk about the game-changer: artificial intelligence. In the age of AI, data quality management is getting a serious upgrade. Machine learning algorithms can sift through oceans of data, spotting patterns humans might miss and flagging inconsistencies faster than you can say “data discrepancy.”
AI-driven tools not only enhance data accuracy by identifying and correcting errors in real-time, but they also bring consistency to the forefront. By learning from past data interactions, AI systems can predict and prevent future errors, ensuring your data remains reliable.
Moreover, these technologies empower predictive analytics, helping businesses forecast trends and make smarter decisions based on high-quality data. AI doesn’t just keep your data clean—it makes it smarter, enabling a level of precision and speed that was previously unattainable.
In short, embracing AI and machine learning in data quality management is like having a supercharged assistant, tirelessly working to maintain the gold standard of data integrity. It’s not just about keeping pace with the future; it’s about defining it, ensuring your data is always ready to support your strategic goals.
[ad_2]