Navigating The World Of Causal Forecasting
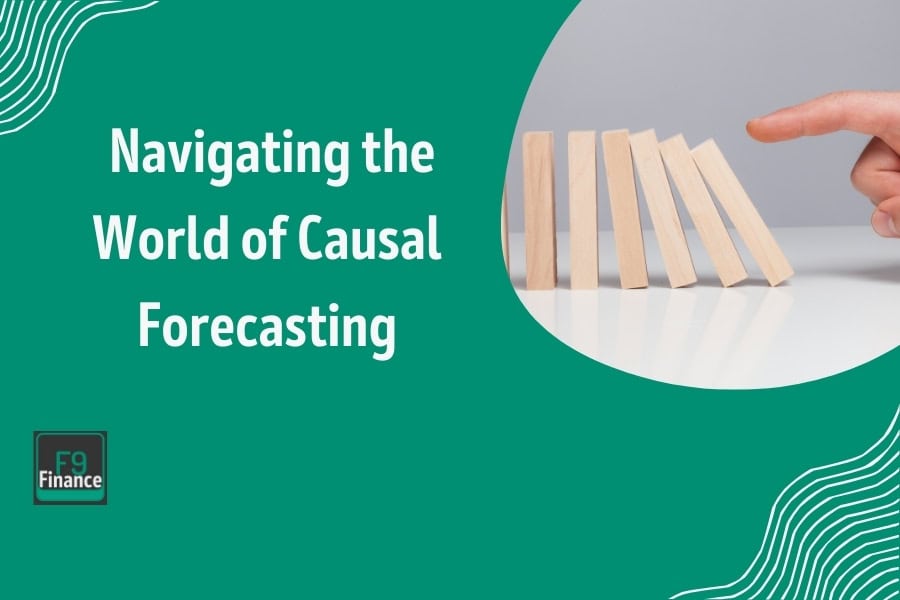
[ad_1]
So, what is causal forecasting, you ask?
Imagine you’re a detective in the finance world, piecing together clues to solve the puzzle of business outcomes. Causal forecasting is all about connecting the dots between cause and effect.
Unlike time-series analysis, which is all about reading patterns over time, causal forecasting digs deeper. It’s like moving from a surface-level weather report to a full-blown meteorological analysis. You’re not just seeing the “what,” but also the “why.” Identifying causal factors is crucial in causal forecasting as it helps in understanding the complex relationships that influence demand, thereby improving forecast accuracy and making informed supply chain decisions.
The Insider’s Advantage
Now, here’s where it gets really interesting. Understanding causal relationships in business is like having a crystal ball that not only predicts the future but also explains why things happen. This insight empowers you to make smarter, more strategic decisions.
By grasping these causal links, you can anticipate market movements, optimize your operations, and even outsmart the competition. It’s a game-changer for strategic planning, turning complex data into clear, actionable insights.
Understanding the Basics of Causal Forecasting
Causal Relationships 101

Let’s start with the basics: what exactly is a causal relationship? Think of it as a direct link between two events, where one event (the cause) directly affects the other (the effect). For example, if I water my plants, they grow.
Simple, right?
But here’s where it gets tricky—correlation versus causation. Just because two things happen together doesn’t mean one caused the other. For instance, ice cream sales and sunburn incidents both rise in summer, but ice cream doesn’t cause sunburns. It’s crucial to distinguish between the two to avoid misleading conclusions. Causal models help in this distinction by using sophisticated analytical techniques to identify true cause-and-effect relationships, improving forecasting accuracy and understanding market dynamics.
Data Sources and Reliability
Next up, sourcing reliable data is like finding a trustworthy friend in the finance world. Accurate data is the backbone of any solid causal analysis. I look for data from reputable sources like government databases, academic research, and trusted industry reports.
Historical data plays a crucial role in building reliable causal forecasts by analyzing existing patterns and trends from past performance. But it’s not just about where the data comes from; data accuracy and quality are paramount. Clean, accurate data ensures that the conclusions I draw are valid and actionable, avoiding the classic “garbage in, garbage out” dilemma.
The Role of Variables
Now, let’s talk about variables. In causal forecasting, we deal with two main types: dependent and independent variables. The independent variable is the one you manipulate or believe to be the cause, while the dependent variable is what you observe or expect to change as a result.
For example, if I’m looking at how advertising spend affects sales, the ad spend is the independent variable, and sales are the dependent variable. Understanding how these variables interact helps me map out the cause-and-effect relationships that are central to accurate forecasting. Additionally, external factors such as commodity prices, competitive activity, and consumer sentiment can significantly impact both dependent and independent variables, thereby influencing the overall accuracy of demand forecasting.
Key Components of Causal Forecasting
Essential Elements of Causal Forecasting
Diving into causal forecasting starts with understanding its crucial building blocks. First up is data collection. It’s the foundation of any forecasting model. Gathering accurate and relevant data is like laying down a robust framework for a skyscraper—it supports everything else.
Next, we have hypothesis testing. Here, I put on my detective hat and start questioning the relationships between variables. Is X really causing Y, or is there another player in the mix? This is where causal inference comes into play, validating the relationships between variables by measuring the impact of various factors, such as macroeconomic events and concurrent influences, on demand. Finally, model selection is where the magic happens. Choosing the right model is akin to picking the right tool for the job. Whether it’s a hammer or a scalpel, the choice can make or break your results.
Causal Models and Techniques

When it comes to modeling techniques in causal forecasting, there are several options in my toolkit. One of the most popular is regression analysis. It’s like the Swiss army knife of modeling—versatile and reliable. Regression helps me understand and quantify relationships between variables, giving a clearer picture of causation.
Causal forecasting works by combining various data sources and analytics to enhance consumer demand predictions, particularly in the retail sector. This multi-faceted approach refines inventory management, improves forecast reliability, and provides actionable insights through deeper market understanding.
But it’s not the only player in town. Techniques like structural equation modeling and causal trees can also provide valuable insights, each with its own strengths and applications. These techniques allow me to tailor my approach, ensuring the model fits the data and the business context like a glove.
Using Indicators and Metrics
Now, let’s talk about indicators and metrics—the GPS of forecasting. Selecting the right key performance indicators (KPIs) is crucial. They’re not just numbers; they’re the pulse of your business strategy.
Understanding future demand is crucial for effective forecasting, as it helps in predicting demand based on the relationships between various independent variables and their impact on inventory, marketing, and pricing strategies. I focus on picking KPIs that align with the goals of the model and the questions I’m trying to answer. It’s about finding those metrics that provide actionable insights, not just noise. Once selected, these indicators guide the forecasting process, helping me track performance and adjust strategies as needed. It’s all about making informed decisions with confidence and clarity.
Step-by-Step Process of Causal Forecasting
Step 1: Define the Problem
Every great journey begins with a clear destination. In causal forecasting, that means nailing down the problem or question you’re tackling. Is it unraveling why sales dip every winter, or understanding the impact of a new marketing strategy? Understanding consumer demand is crucial here, as macroeconomic factors like tax refunds and daylight saving time changes can significantly affect it. Pinpointing the issue helps me set the stage for effective analysis and ensures I’m not barking up the wrong tree.
Step 2: Gather and Prepare Historical Data
Next, it’s time to roll up my sleeves and dive into data collection. The goal is to gather relevant information from credible sources. But raw data is messy, so I employ strategies like cleaning and organizing to whip it into shape. Tools like Excel, R, or Python become my best friends here, streamlining the process and ensuring I’m working with the most accurate dataset possible. Additionally, leveraging machine learning can enhance data preparation and analysis by uncovering intricate patterns and underlying demand signals that traditional methods might miss.
Step 3: Identify Causal Relationships
With clean data in hand, I start searching for those elusive causal links. It’s a bit like detective work, piecing together clues to discern which variables are playing the lead roles. Identifying causal factors is crucial for understanding the complex relationships that influence demand and improve forecast accuracy. Data visualization tools and statistical tests help me validate these relationships, making sure I’m not mistaking mere correlation for true causation.
Step 4: Build the Model
Now, it’s showtime! I build my causal model by selecting the right variables and structuring them to reflect the real-world scenario I’m analyzing. Causal models play a crucial role in building accurate forecasts by leveraging technology and data analytics to understand market dynamics and customer behavior. Whether it’s using regression analysis or more advanced techniques, this step is about creating a blueprint that accurately represents the causal dynamics at play.
Step 5: Test and Validate the Model
No model is complete without rigorous testing. I put my model through its paces, using techniques like cross-validation to check its accuracy and reliability. Additionally, causal inference methods are employed to validate the model by measuring the impact of various factors, such as macroeconomic events and concurrent influences, on demand. This step is crucial for ensuring that my model isn’t just a theoretical construct but a practical tool that can stand up to the unpredictability of the real world.
Step 6: Implement and Monitor
Finally, I take my model out of the lab and into the wild. Implementing it in real-world scenarios allows me to see if it truly delivers on its promises. Demand planning plays a crucial role in this phase, as it helps in refining forecasting methods and optimizing inventory management through data-driven insights. But the work doesn’t stop there—I continuously monitor its performance, ready to tweak and adapt as necessary to ensure it remains relevant and effective.
Causal Forecasting Case Studies
Success Stories
Let’s kick things off with the wins.
One of my favorite success stories involves a retail giant that used causal forecasting to reshape its inventory management. By identifying the key drivers behind customer demand, they optimized stock levels, which not only cut down on excess inventory but also boosted sales during peak seasons. Understanding future demand allowed them to anticipate market needs and adjust their strategies accordingly.
Another standout is a tech company that leveraged causal insights to fine-tune their marketing campaigns. By understanding which promotional efforts truly drove sales, they increased their ROI and outpaced competitors. These companies didn’t just predict the future—they shaped it.
Learning from Mistakes
Of course, not every story in the world of causal forecasting ends in triumph.
Take the case of a beverage brand that misinterpreted the data, mistaking a seasonal spike as a year-round trend. They ramped up production based on flawed predictions, leading to significant overstock and financial loss. Ignoring external factors such as competitive activity and consumer sentiment contributed to these flawed predictions. The lesson here? Ensure robust data validation and a deep understanding of market dynamics.
Another cautionary tale is a startup that placed too much faith in a single variable, ignoring broader market trends. It serves as a reminder to always consider multiple factors and continuously re-evaluate assumptions. Mistakes are part of the journey, offering valuable lessons that refine our forecasting prowess.
Common Challenges and Solutions in Causal Forecasting
Data Limitations
Ah, data—the lifeblood of causal forecasting, yet often the source of many headaches. One of the biggest challenges I face is dealing with incomplete or inconsistent data. It’s like trying to assemble a puzzle with missing pieces.
To combat this, I prioritize data cleaning and validation, ensuring the information I use is as accurate and complete as possible. When data gaps persist, I use statistical methods like imputation to fill in the blanks without compromising the integrity of the analysis. Additionally, historical data can be leveraged to address these limitations by analyzing existing patterns and trends from past performance. It’s all about making the most of what I have and being resourceful in the face of limitations.
Interpreting Results
Once I’ve crunched the numbers, the next hurdle is interpreting the results. It’s easy to fall into the trap of overconfidence, mistaking correlation for causation.
Understanding causal factors is essential in this process, as it helps identify and comprehend the complex relationships that influence demand, thereby improving forecast accuracy and making informed supply chain decisions.
My tip?
Always question the results and consider alternative explanations. Visualization tools can be incredibly helpful here, allowing me to see patterns and relationships more clearly. It’s also crucial to remember that forecasts are just models—use them as guides, not absolute truths. By maintaining a healthy skepticism and seeking peer feedback, I can ensure my interpretations are both accurate and actionable.
Dealing with Uncertainty and Market Dynamics
Let’s face it: uncertainty is an unavoidable part of forecasting. But instead of fearing it, I embrace it as part of the process. One strategy I use is scenario analysis, which involves creating multiple forecasts based on different assumptions. This helps me understand the range of possible outcomes and prepare for various scenarios.
Additionally, I continuously update my models as new data becomes available, allowing me to adjust forecasts and stay responsive to changing conditions. Businesses should embrace causal forecasting to navigate uncertainties, as it offers a flexible and adaptive approach to achieving more accurate and actionable forecasts tailored to their specific needs. In the world of causal forecasting, flexibility and adaptability are key to navigating the unknown.
Tools and Software for Causal Forecasting
Choosing the Right Tools
Navigating the world of causal forecasting can feel like shopping for the perfect pair of shoes—so many options, but only a few that truly fit.
Some of my go-to tools include R and Python for their robust statistical packages, Tableau for its stellar data visualization capabilities, and Microsoft Excel for its unparalleled accessibility. Selecting the right causal models is crucial for accurate forecasting, as they help in understanding market dynamics and improving forecasting accuracy.
Each tool brings something unique to the table, whether it’s advanced analytics or user-friendly interfaces. The key is to match the tool with the project’s specific needs and the team’s expertise.
Pros and Cons
Every tool has its charm and its quirks. R and Python, for instance, are powerhouses for statistical analysis, but they have a steeper learning curve and require a bit of coding savvy. On the flip side, Excel is incredibly intuitive, allowing for quick forecasts even for those who might not be data wizards.
However, its scalability can be a limitation for large datasets. Tableau shines in making complex data stories visually engaging, but comes with a price tag that can be a hurdle for smaller businesses. It’s all about weighing these strengths and weaknesses to find the best fit for the task at hand. Effective demand planning plays a crucial role in this process, as it helps in selecting tools that enhance forecasting methods and optimize inventory management strategies.
Integration and Implementation
Getting a new tool to play nicely with existing systems can be a challenge, but it’s far from impossible. Start by ensuring compatibility between the new software and your current systems—this might involve updates or setting up APIs to facilitate data flow. Integrating causal forecasting techniques into existing systems can further enhance the understanding of consumer behavior and market dynamics by isolating actual demand signals from market noise.
Training is crucial; even the best tool is useless if the team doesn’t know how to wield it effectively. I also recommend piloting the tool with a smaller project before full-scale implementation to iron out any kinks. With the right preparation and a bit of patience, integrating these tools can enhance forecasting capabilities and drive data-driven decision-making to new heights.
[ad_2]